Revolutionizing AI: MILS and the Rise of Open-Source Solutions
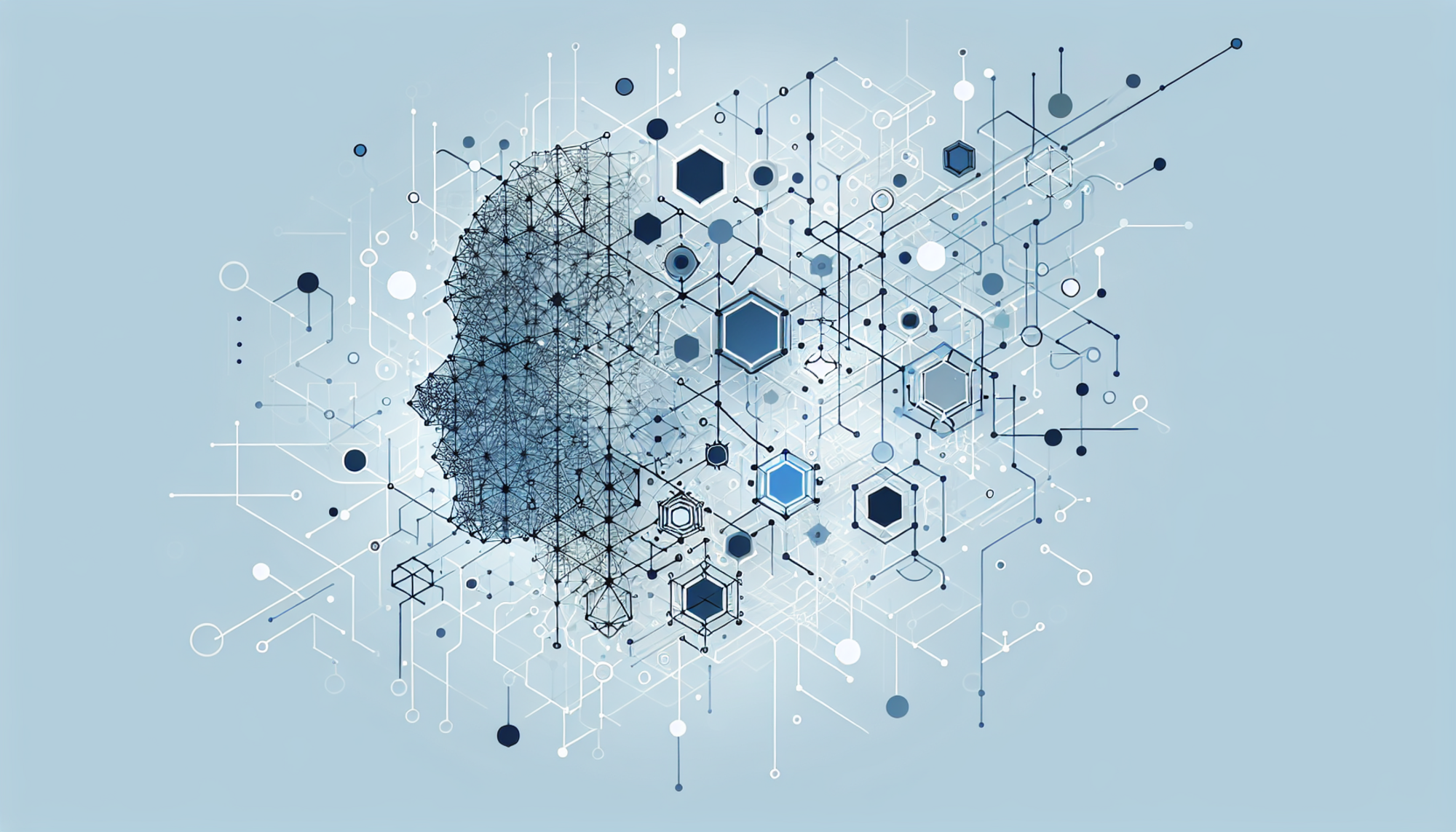
In an AI world where innovation seems to spring forth faster than you'd expect at a caffeine-fueled hackathon, two recent articles from MarkTechPost have taken the spotlight. The first, titled "Meta AI Introduces MILS: A Training-Free Multimodal AI Framework for Zero-Shot Image, Video, and Audio Understanding", explores the cutting-edge framework MILS (Multimodal Iterative LLM Solver). The second piece titled "4 Open-Source Alternatives to OpenAI’s $200/Month Deep Research AI Agent" reveals powerful open-source alternatives to OpenAI's costly deep research assistant. Let’s dive into how these posts educate us on today's AI advancements and explore the broader implications for developers and researchers alike.
Breaking Down MILS: An AI Marvel
The MILS framework presents a groundbreaking approach to multimodal reasoning—a domain where traditional LLMs often falter. As the article details, MILS operates on the innovative premise that AI can work effectively across various content types (images, audio, text) without the burdensome need for task-specific training. Instead, it utilizes an iterative optimization cycle involving a GENERATOR and a SCORER, enhancing the output in real-time.
This method stands out vividly amid traditional approaches that link performance strictly to extensive datasets and laborious training protocols. As noted, by using pre-trained models within an adaptive feedback loop, MILS opens doors to zero-shot generalization applicable to diverse tasks. This development not only makes the framework versatile but also paves the way for democratizing access to advanced multimodal capabilities, bridging tech barriers for small developers.
Cost-Efficiency: The Open Source Revolution
While MILS showcases a remarkable leap forward, the second article highlights the burgeoning landscape of open-source alternatives, particularly against a backdrop of rising costs associated with proprietary tools. The alternatives listed—Deep-Research, OpenDeepResearcher, Open Deep Research by Firecrawl, and DeepResearch by Jina AI—provide robust, customizable options that promise to strip away the high price tag while still delivering powerful performance.
As the piece astutely points out, these alternatives rely on community-driven innovation, enabling users to tailor functionalities to meet specific research needs. The potential for enhanced accessibility is clear: researchers from universities to startups can now engage in deep explorations without breaking the bank. With fully open-source options available, we witness a gradual shift from proprietary to community-powered solutions—a pattern likely to gain momentum in the AI ecosystem.
Building the Future: Common Threads
A common theme in both pieces is the emphasis on accessibility and the breaking down of barriers within the AI research community. MILS offers a promising direction for multimodal output without the hefty training overhead, while the open-source alternatives democratize access to sophisticated AI research assistants. Both narratives lead to a future where effective tools are within the reach of not just the well-funded, but also individual developers and smaller organizations.
Moreover, the integration of advanced models without extensive configurations sounds like music to the ears of programmers looking for swift and efficient implementations. Such developments suggest a trend towards more developer-friendly environments in a domain often criticized for its complexity. The message is clear: innovation should empower, not enchain.
The Takeaway: Your Next Steps
As we digest this wealth of knowledge, it's crucial to consider what this means for individual developers and teams. The advancement of MILS could directly influence how AI applications are designed—prioritizing multimodal capabilities without the typical hassle. This offers an opportunity to innovate in areas that were once restricted by cost and complexity.
On the other hand, the emergence of open-source options encourages exploration and experimentation. Developers can prototype ideas without heavy infrastructure investments. These changes advocate a more resilient and adaptable AI landscape, ideally suited for rapid iteration and collaboration in projects ranging from academic research to industry ventures.
Final Thoughts: Embracing Change
In conclusion, both articles from MarkTechPost serve as excellent indicators of the shifting paradigms in AI development. With innovations like MILS, alongside powerful open-source alternatives, the future seems not just bright, but also incredibly promising for diverse teams looking to harness the power of AI. So here's hoping all developers, researchers, and enthusiasts embrace these changes and keep pushing the boundaries of what’s possible in AI!