AI Innovations: From Periodic Tables to Hedge Fund Strategies
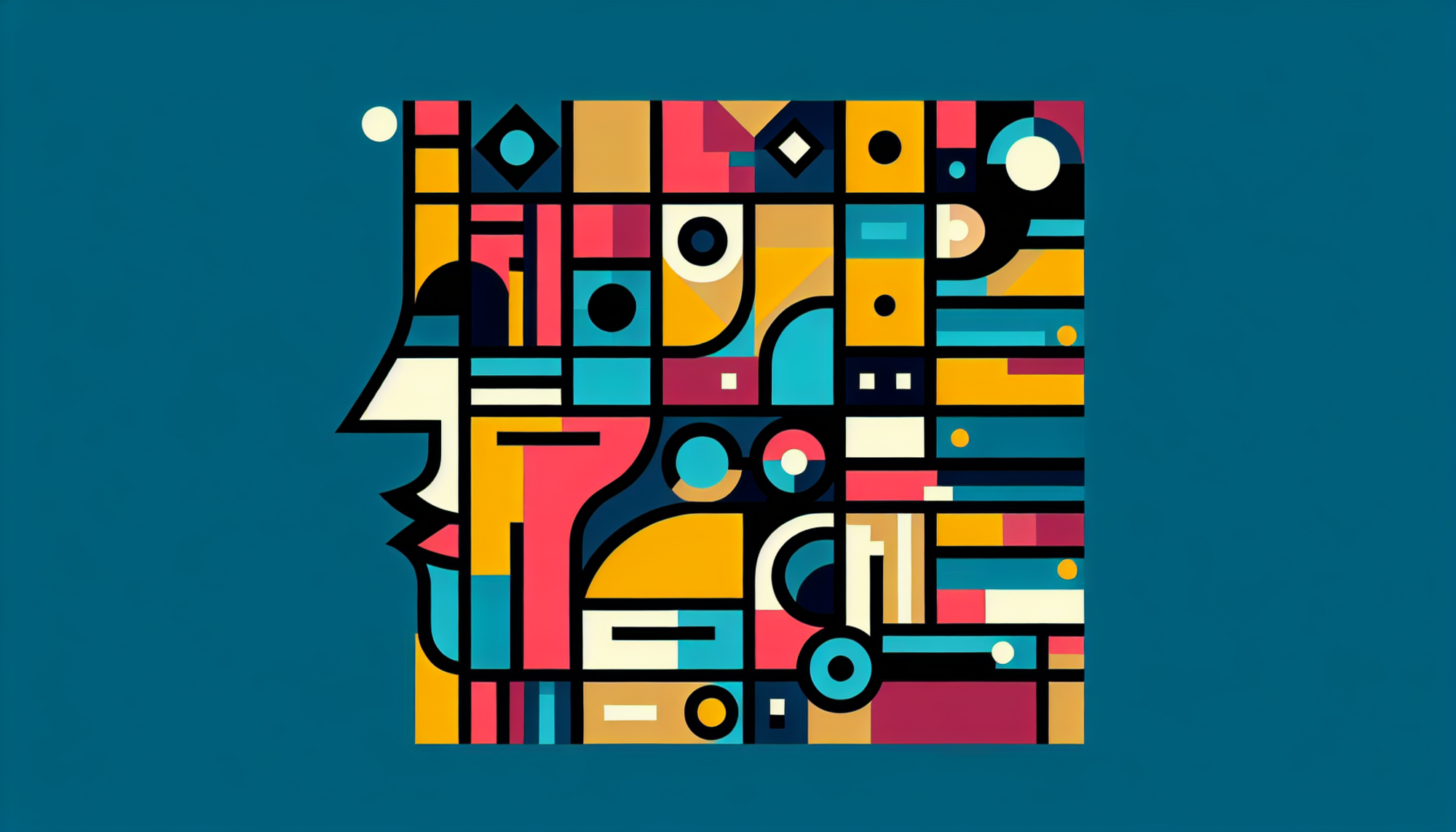
In the vast and ever-evolving landscape of artificial intelligence (AI), recent contributions highlight both the innovative potential and the economic implications of machine learning. From pioneering frameworks guiding algorithm design to the impact of AI on finance, these various perspectives coalesce to paint a nuanced picture of AI's future. The collection of blog posts explored delves into topics such as the "periodic table of machine learning," the intricacies of Gemini 2.5 Flash, and the employment of AI in hedge funds, providing a broad understanding of how AI is shaping our world.
Periodic Table of Machine Learning: A Novel Framework
One of the standout developments discussed in the recent MIT article is the introduction of a "periodic table of machine learning". This groundbreaking work organizes over 20 classical algorithms into a cohesive framework, much like the chemical periodic table. The unification of algorithms serves as a powerful tool for researchers, allowing for the transfer of insights across different algorithms and inspiring the development of new, improved versions. The creators have not only categorized these algorithms but have identified gaps, pointing towards undiscovered possibilities in AI research (Zewe, 2025).
According to Shaden Alshammari, a lead researcher in the project, this structure allows for systematic exploration rather than chaotic experimentation. This structured approach could potentially accelerate algorithm discovery and enhance predictive capabilities across various applications, thereby revealing the interconnected pathways of AI algorithms (Zewe, 2025).
Gemini 2.5 Flash: Bridging Speed and Complexity
In a different realm, DeepMind has launched Gemini 2.5 Flash, a hybrid reasoning model that emphasizes both speed and decision-making finesse. This model allows developers to actively manage "thinking budgets", striking a balance between computational cost and output quality. By introducing the concept of controlled reasoning, Gemini provides an innovative solution to complex problem-solving scenarios, potentially outclassing its forerunners (Doshi, 2025).
The ability to toggle reasoning capabilities presents developers with a unique advantage: speeding up simple queries while diving deeper into complex problems when necessary. This kind of flexibility marks a significant evolution in AI performance and usability. It raises intriguing questions about the future of AI capabilities and the ethical considerations surrounding such powerful tools (Doshi, 2025).
AI's Role in Economic Landscapes
Another noteworthy blog elaborates on how hedge funds are leveraging AI technology to maintain competitive advantages in volatile markets. The applications of AI in this domain range from predictive analytics to real-time decision-making strategies. Hedge funds, by incorporating sophisticated AI mechanisms, can use vast datasets to forecast market trends, highlighting a shift towards a data-driven culture (Davis, 2025).
However, such reliance on AI poses ethical dilemmas and risks. The opacity of AI decision-making processes raises concerns surrounding market manipulation and the necessity for transparent frameworks. The future of hedge funds may well hinge on striking a delicate balance between machine efficiency and human oversight, ensuring that any advancement serves all stakeholders equitably (Davis, 2025).
The Positive and Negative Aspects of AI Integration
The integration of AI into sectors like cryptocurrency trading also reflects broader implications of AI technology. While AI offers unprecedented efficiency and speed, it equally introduces risks such as market instability and ethical quandaries arising from its opaque functioning. Investors and legislators must grapple with the implications of rapid technological adoption and the potential for market manipulation (Davis, 2025).
Moreover, the ability for novice traders to access complex trading mechanisms via AI has redefining implications, potentially democratizing finance while simultaneously posing risks for uninformed investors. The evolving relationship between AI and economic factors is complex and will continue to grow as technologies advance.
Conclusion: The Path Forward for AI
The development of frameworks like the periodic table of machine learning and advancements like Gemini 2.5 Flash signal an exciting yet cautionary journey into the future of AI. As society grapples with the rapid integration of AI across numerous domains, ethical considerations and the necessity for human oversight remain paramount. Moving forward, it will be essential to nurture innovation while actively addressing the implications of these technologies on diverse economic landscapes.
References
- Zewe, A. (2025). "Periodic table of machine learning" could fuel AI discovery. MIT News.
- Doshi, T. (2025). Start building with Gemini 2.5 Flash - Google Developers Blog.
- Davis, E. (2025). How Hedge Funds Leverage AI for Competitive Advantage. AI2People.
- Davis, E. (2025). AI in Cryptocurrency Trading: Boon or Bane?. AI2People.